Speakers 2025
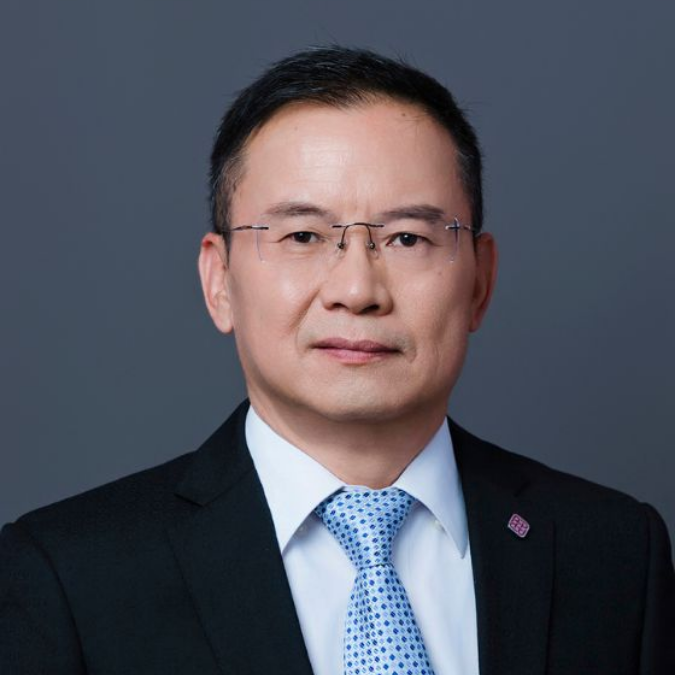
Prof. Qing Li
Hong Kong Polytechnic University, China
Fellow of IEEE, AAIA, and IET/IEE
Biography: Qing Li is a Chair Professor and Head of the Department of Computing, the Hong Kong Polytechnic University. He received his B.Eng. from Hunan University (Changsha), and M.Sc. and Ph.D. degrees from the University of Southern California (Los Angeles), all in computer science. His research interests include multi-modal data management, conceptual data modeling, social media, Web services, and e-learning systems. He has authored/co-authored over 500 publications in these areas, with over 42,000 citations and H-index of 84 (source: Google Scholars). He is actively involved in the research community and has served as Editor-in-Chief of Computer & Education: X Realitty (CEXR) by Elsevier, associate editor of IEEE Transactions on Artificial Intelligence (TAI), IEEE Transactions on Cognitive and Developmental Systems (TCDS), IEEE Transactions on Knowledge and Data Engineering (TKDE), ACM Transactions on Internet Technology (TOIT), Data Science and Engineering (DSE), and World Wide Web (WWW) Journal, in addition to being a Conference and Program Chair/Co-Chair of numerous major international conferences. He also sits/sat in the Steering Committees of DASFAA, ER, ACM RecSys, IEEE U-MEDIA, and ICWL. Prof. Li is a Fellow of IEEE, AAIA, and IET/IEE.
Speech Title: KCUBE - A Knowledge Graph University Curriculum Framework for Student Advising and Career Planning
Abstract: Knowledge representations and interactions are at the forefront of teaching, learning, and career planning activities in all endeavors of education and career development. University students are increasingly faced with a myriad of interdisciplinary topics that are seemingly unrelated when unstructured knowledge representations are presented, especially during advising and career orientation sessions. This is especially challenging in fast-changing technical domains such as Computer and Data Science where university curricula are reviewed on an annual basis. This makes it increasingly difficult for instructors and administrators to present both the big picture as well as the detailed knowledge components of degree programs to students when choosing a career or establishing a plan of study and assessment. This paper introduces the KCUBE project, a virtual reality knowledge graph framework for structuring and presenting both the overall view of the Computer Science curriculum taught in the Department of Computing at the Hong Kong Polytechnic University as well as the scheduling alternatives in managing course content and presentation views by instructors and students. We employ computational information storage and retrieval methods, machine learning, and interactive virtual reality to better understand, manipulate, and visualize abstract concepts and relationships in the development of teaching and learning activities in our department.
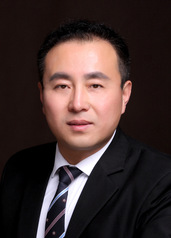
Prof. Guangjie Han
Hohai University, China
Biography: Guangjie Han is a professor, currently serving as the Dean of the School of Information Science and Engineering at Hohai University. He is an IEEE Fellow, IET/IEE Fellow, and AAIA Fellow. His main research interests include smart oceans, industrial IoT, artificial intelligence, networks, and security. In recent years, he has published more than 350 high-level SCI journal papers, including over 130 papers in the IEEE/ACM Trans. series, in international journals such as IEEE JSAC, IEEE TMC, IEEE TPDS, and IEEE TCC. His publications have been cited over 20000 times on Google Scholar, with an H-index of 75. He has authored three monographs and translated one book. He has led more than 30 provincial and ministerial-level research projects, including national key R&D programs and national natural science foundation key projects. He has been granted 130 national invention patents and 6 PCT international authorized patents. He has received numerous awards, including the second prize of the China Business Federation Science and Technology Award, the third prize of the Jiangsu Provincial Science and Technology Award, the second prize of the Liaoning Provincial Science and Technology Progress Award, and the Best Paper Award of the IEEE Systems Journal in 2020. For six consecutive years (2019-2024), he has been listed as one of the top 2% of scientists globally, as well as for the Chinese Highly Cited Researchers list for five consecutive years (2020-2024). Currently, he serves as an associate editor for more than ten international journals, including IEEE TII, IEEE TCCN, and IEEE Systems. He has been awarded the "333 High-level Talents in Jiangsu Province" (second level), the "Outstanding Contribution Young and Middle-aged Experts in Jiangsu Province," the "Minjiang Scholar Lecture Professor," and the "May 1st Labor Medal" of Changzhou City.
Speech Title: Software Re-define the Underwater Multi-Agent Networks: Architecture, Key technologies, and Applications
Abstract: The complexity of the marine environment has become a key factor restricting the development of underwater multi-agent intelligence technology. For this, this report introduces the concept of underwater multi-agent network. On this basis, the "software-defined network" technology is introduced to reconstruct the infrastructure of the underwater multi-agent network. Then, combined with the research results of our team, this report introduces how to use software-defined network technology to reconstruct the data routing architecture, formation navigation architecture and autonomous learning decision-making architecture of the underwater multi-agent network. This report aims to provide a research idea, re-examine the collaborative control mechanism of underwater multi-agent systems, and build a "software-defined smart ocean".
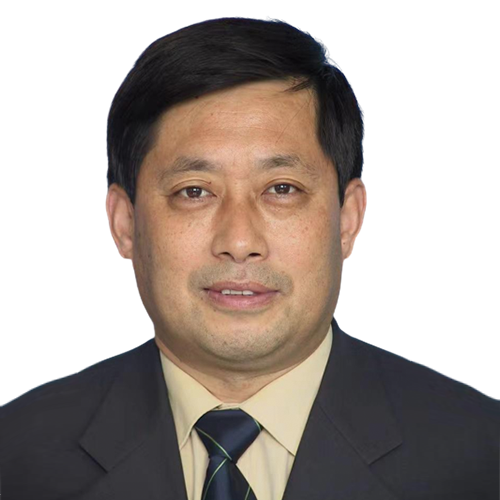
Prof. Chengdong Wu
Northeastern University, China
Biography: Professor Wu got the M.Sc. degree in Automatic Control Theory and Application from the Tsinghua University, China, 1988. He got the Ph.D. in Industrial Automation from the NEU, China, 1994. He finished the Post-Doctoral Research from the University of Wales, UK, 1997. He has finished more than of 30 research projects funded by the Chinese government and industrial fields. About 300 of journal and conference papers and 9 books have been published. 13 research prizes and teaching prizes were awarded from the Chinese government. His main research interesting is included machine vision, image processing, robot control, and machine learning.
Speech Title: Research and Application of Construction Robotics Technology
Abstract: Construction robots constitute a pivotal research direction within the field of robotics. This report delves into the progress of research and application of construction robotics technology both domestically and internationally. It analyzes the demands and characteristics of the industry for construction robotics technology. Drawing on typical cases, including bricklaying robots, 3D printing robots, steel tying robots, demolition and cleanup robots, inspection robots, humanoid robots, wearable robotic devices, transportation robots, as well as the utilization of unmanned aerial vehicles (UAVs) in the construction sector, the report presents advancements in the research of construction robotics technology. Furthermore, it discusses technical features such as construction robot control, environmental perception and modeling, human-robot collaboration, and explores the ways in which artificial intelligence technology can empower intelligent construction robots. Lastly, the report offers insights into the future development trends of construction robotics technology.
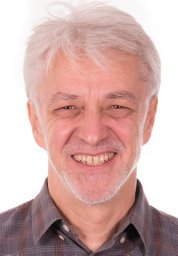
Prof. Vladan Devedzic
University of Belgrade, Serbia
Biography: Vladan Devedzic is a Professor of Computer Science and Software Engineering at the University of Belgrade, Faculty of Organizational Sciences. He is the founder and head of the research group focused on Artificial Intelligence (GOOD OLD AI research network). He is also the founder of the Artificial Intelligence Laboratory at his home faculty. Since 2021, he has been a corresponding member of the Serbian Academy of Sciences and Arts (SASA) at the Department of Technical Sciences. Vladan Devedžić's continuous professional goal is to bring together ideas from the field of Artificial Intelligence / Intelligent Systems and Software Engineering. His current interests include artificial intelligence, programming education, software engineering, intelligent software systems, and technology-enhanced learning. He has authored/co-authored numerous research papers, published in international and national journals and edited books, or presented at international and national conferences. He has also authored/co-authored six books on intelligent systems and software engineering. He has also given more than 20 invited talks, keynote talks and plenary talks at international conferences, as well as more than 20 tutorials. His international research cooperation and collaboration record includes universities and research institutes from EU, USA, Canada, Japan, Australia, New Zealand, India and China. He received the Annual Award of the University of Belgrade for an outstanding research contribution (2006) and the award for one of the three best projects in the field of educational technologies at the annual Online Educa Berlin meeting, Berlin (2015).
Speech Title: "We don't need no education"? Careful with this when it comes to AI!
Abstract: Today’s industry is hungry for AI skills. This industry is fast-paced and is more and more data-driven, hence a strong grasp of AI technologies is vital for career success. Moreover, the extremely high dynamics of AI technologies brings the need for new AI skills and for professionals to fill new AI jobs, skills and jobs that did not exist until very recently; here we are talking about months, not years. Examples include LLMOps (Large Language Model Operations, i.e. the practices and processes involved in managing and operating LLMs); creating synthetic content – text, images, video, audio and data – using generative AI tools; AI ethics and governance (ensuring that AI technology is used ethically, focusing on accountability, fairness, transparency, and compliance with new regulations); LMM engineering (Large Multimodal Model development and deployment, combining multiple data modalities (text, images, audio, video); and many more.
This rapidly evolving landscape of new AI skills and jobs demands a fundamental shift in educational approaches across all levels. This talk contributes to the ongoing debate on how to effectively integrate AI in education at schools and universities. Key considerations include:
• Defining the essential foundational knowledge required for students at each educational stage to grasp AI concepts.
• Determining the optimal balance between theoretical instruction, practical applications (including real-world AI examples and student-led AI projects), and critical discussions on the potential misuse and abuse of AI.
• Addressing the broader societal implications of AI's rapid advancement, with a focus on identifying and mitigating the most significant risks and threats.
It is important to stress that the talk raises many open questions. There are no definite answers, and all answers are typically short-lived. The attendees are welcome to actively participate in the talk.
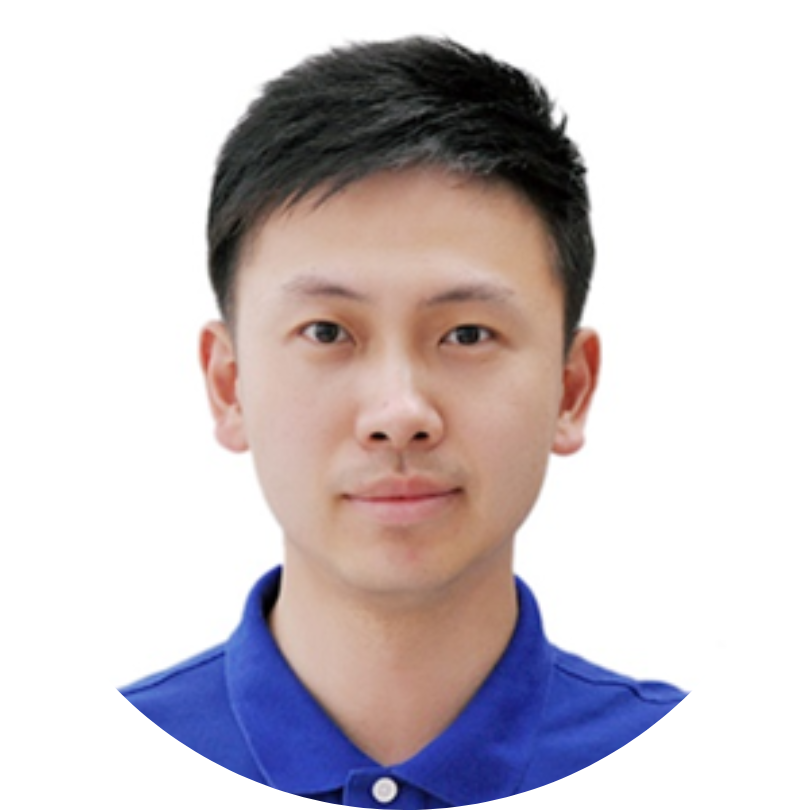
Prof. Xianglei Xing
Harbin Engineering University, China
Biography: Xianglei Xing is a Professor and Ph.D. supervisor at Harbin Engineering University. He has also served as a visiting researcher at the University of California, Los Angeles (UCLA). Currently, he is the Director of Research at the Institute of Intelligent Systems from the College of Intelligent Systems Science and Engineering and serves as an editorial board member of the CAAI Transactions on Intelligent Systems.
Professor Xing’s long-term research interests lie in the fields of Artificial General Intelligence (AGI) and Maritime Intelligence, focusing on both fundamental theories and practical applications. He has led and participated as a principal investigator in several major research projects, including the Joint Key Funds of the National Natural Science Foundation of China (NSFC), NSFC, the CAAI-Huawei MindSpore Academic Award Fund, and the Defense Science and Technology Administration's Smart Shipping Program.
His academic contributions have been recognized with numerous honors, including the First Prize of the Natural Science Award of Heilongjiang Province for Higher Education Institutions and the Excellent Paper Award from the CAAI Transactions on Intelligent Systems. He has authored one academic monograph and two textbooks under the Ministry of Education's 14th Five-Year Plan for Strategic Emerging Fields. He has published over 60 high-quality research papers in leading journals and conferences such as IEEE Transactions on Pattern Analysis and Machine Intelligence (T-PAMI), Pattern Recognition, IEEE Transactions on Circuits and Systems for Video Technology (T-CSVT), CVPR, ICML, and AAAI, with more than 1,000 citations.
Speech Title: Unifying Appearance-Based and Model-Based Multi-View Gait Recognition through Contextual Spatio-Temporal Features within a Differentiable Rendering Framework
Abstract: Existing appearance-based gait recognition methods excel at extracting rich spatial features of human gait but often neglect the fine-grained temporal dynamics and 3D physical information critical to distinguishing subtle movement patterns. On the other hand, model-based approaches, which rely on 3D human models, are typically learned from general human pose datasets, leading to distribution drift and reduced effectiveness for gait recognition tasks. In this talk, we propose a novel differentiable rendering framework that seamlessly integrates appearance-based and model-based gait recognition methods. This framework combines the structural precision of model-based techniques with the discriminative power of appearance-based methods, enabling robust gait recognition across a wide range of conditions.
Recognizing gait as a fine-grained recognition challenge, we emphasize the uniqueness of individual movement patterns across different temporal sequences. To address this, we introduce a local multi-scale and global contextual spatio-temporal network tailored for gait recognition. This network divides the gait sequence into sub-sequences, each with varying spatial resolutions, and captures multi-scale temporal features. Temporal context is extracted using a transformer model, which integrates the contextual information from the sub-sequences. These sub-sequences are then fused to generate a comprehensive global feature representation, enhancing recognition accuracy and robustness. Through this framework, we provide a unified approach that leverages both appearance-based and model-based strategies, ultimately improving gait recognition performance, particularly in multi-view and dynamic environments.